Document Type
Article
Keywords
affinity analysis; association rules; adaptive DM development cycle; decision trees; Inductive inference; student retention
Publisher
International Journal of Computer Science
Rights Management
Copyright International Journal of Computer Science
Abstract
Technological development has engaged educational institutions in fierce global competition. To be competitive in meeting the changing needs of today’s student population, educational institutions find it imperative to prioritize student retention efforts and to develop strategies that interact with students to effectively provide additional value and service. In this study we developed a two-module system: a decision tree for predicting a student’s decision to stay until graduation and an affinity analysis algorithm for identifying the relationship between student attributes and student decisions. We followed a three-phase-six-stage adaptive data mining cycle in developing a knowledge base for student retention strategies. The affinity analysis initially identified more than 400 association relationships with student retention. By applying inductive inference, the association rule set was refined iteratively down to less than 30 rules, and useful strategic implications were developed regarding how the selected factors were associated with a student’s decision. This set of implications and factors was then integrated into the development of strategies for student retention.
Included in
Business Analytics Commons, Management Information Systems Commons, Other Business Commons
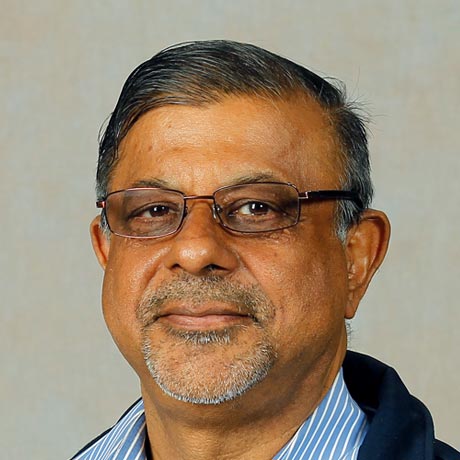